An open source tool that can substitute traditional, labour-intensive methods
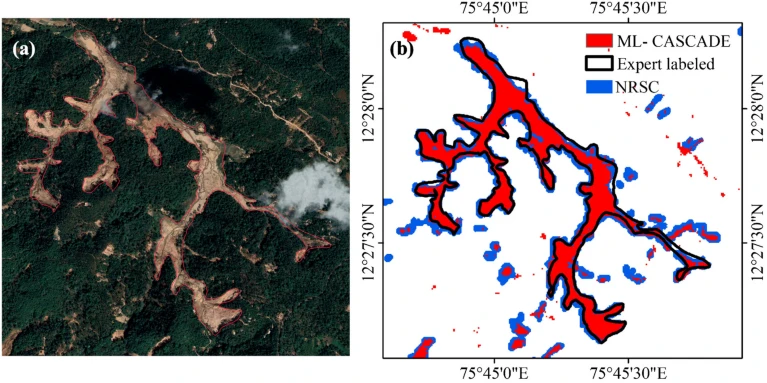
A manual map of landslide extent (left) and the extent as shown by ML-CASCADE (right). Credit: Nirdesh Kumar Sharma, Manabendra Saharia
__________________________________________________________________
Scientists have developed a machine learning and cloud computing-based tool that can map landslide clusters in five minutes and simple events in just two, crucial for improving post-disaster risk and damage assessment1.
Understanding and mitigating landslides is challenging owing to the lack of spatial and temporal data. In a recent study, scientists at the Indian Institute of Technology in Delhi demonstrated how ML-CASCADE can be used to rapidly measure landslides using satellite data.
The semi-automatic method combines satellite data, terrain data, vegetation indices (measures of plant health), and machine learning. It uses pre- and post-event images from the Earth observation Sentinel-2 satellites, along with terrain factors, to classify areas as landslide or non-landslide. The application runs on Google Earth Engine’s cloud computing platform, allowing users to generate landslide maps instantly.
The tool’s effectiveness was demonstrated using two case studies — the Kodagu landslide in Karnataka’s Western Ghats and the Kotrupi landslide in Himachal Pradesh’s Himalayas. For Kodagu, ML- CASCADE produced a precise landslide map in five minutes, which closely matched expert assessments. Similarly, the tool accurately captured the Kotrupi landslide’s extent in under a minute, matching existing manual and semi-automated methods.
ML-CASCADE’s advantages include speed, accessibility, and adaptability to varied terrain, making it suitable for low-resource settings. Its disadvantages are that, it may overestimate areas near riverbanks, and it relies on user-supplied training samples which introduces some subjectivity.
Future work could involve refining the tool’s accuracy in different terrains and integrating it with other disaster management systems.
doi: https://doi.org/10.1038/d44151-024-00178-5
[This research highlight was partially generated using artificial intelligence and edited by a staff member of Nature India.]
References
- Sharma, N. & Saharia, M. Landslides (2024).
source: http://www.nature.com / Nature India / Home> Nature> Nature India> Research Highlights> Article / Ocotober 29th, 2024